The Future of Business: Embracing Machine Learning
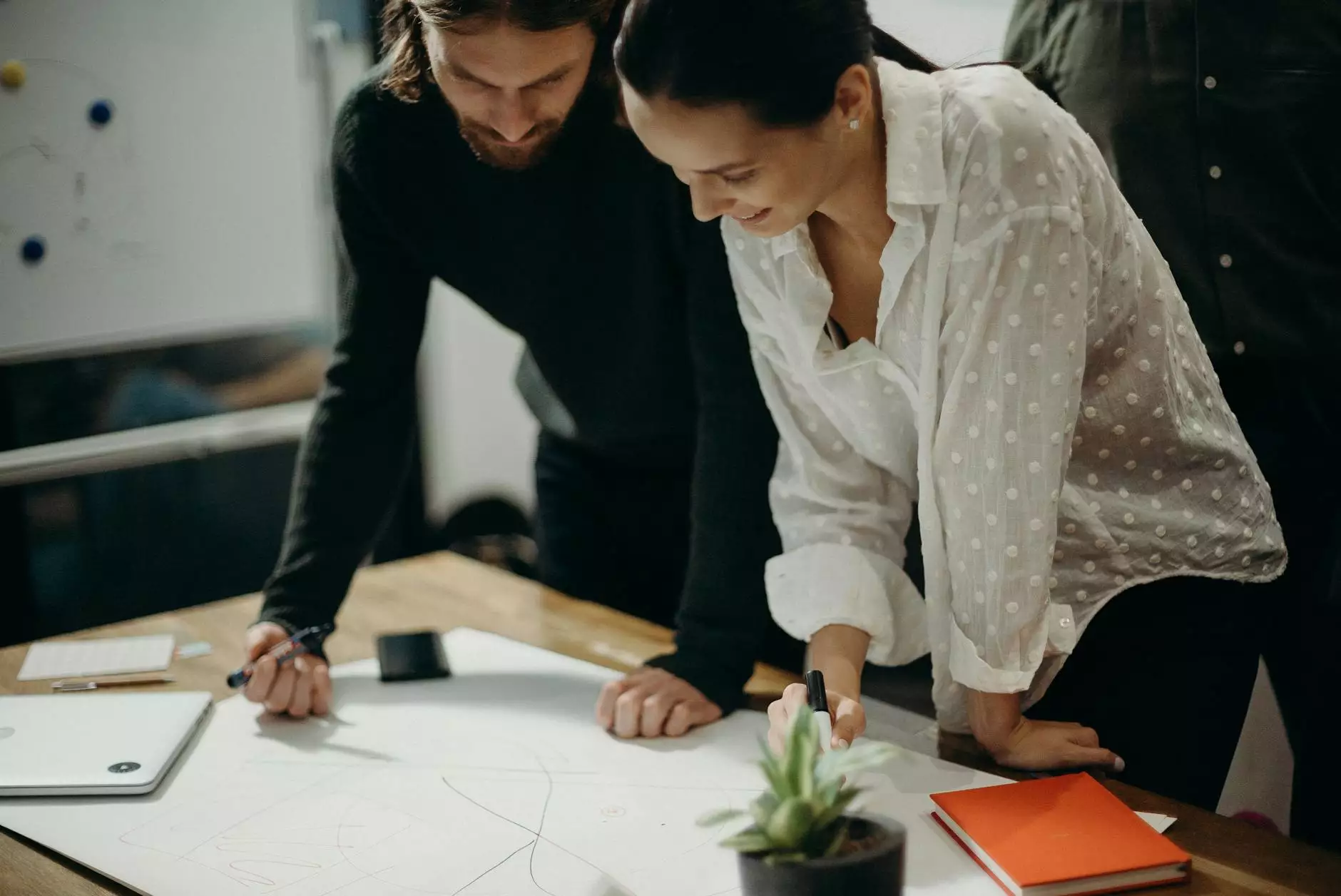
In the contemporary world, machine learning has emerged as a pivotal force reshaping business landscapes. This blog on machine learning delves into how organizations leverage this technological advancement to enhance efficiency, make data-driven decisions, and foster innovation. The ensuing paragraphs elucidate the significance of machine learning, its various applications across industries, and the challenges businesses face as they navigate this transformative journey.
Understanding Machine Learning
Machine learning, a subset of artificial intelligence (AI), involves the development of algorithms that allow computers to learn from and make predictions based on data. Unlike traditional programming, where explicit instructions dictate outcomes, machine learning enables systems to identify patterns and improve their performance over time with minimal human intervention. This capacity not only accelerates processes but also enhances accuracy in decision-making.
The Importance of Machine Learning in Business
In today’s fast-paced business environment, the ability to extract actionable insights from vast amounts of data is crucial. Here are several reasons why machine learning is essential for businesses:
- Data-Driven Decision Making: Machine learning equips organizations to analyze extensive datasets, facilitating informed decisions based on empirical evidence rather than intuition.
- Increased Efficiency: Automating repetitive tasks allows businesses to focus their resources on strategic initiatives, thus fostering innovation and growth.
- Enhanced Customer Experiences: Personalized marketing strategies powered by machine learning can significantly improve customer satisfaction and retention rates.
- Risk Management: Machine learning models can help predict and mitigate risks by providing insights into potential challenges before they arise.
Applications of Machine Learning in Diverse Industries
1. Healthcare
The healthcare industry is experiencing a significant transformation due to machine learning. By analyzing patient data, machine learning algorithms assist in diagnosing diseases, predicting patient outcomes, and personalizing treatment plans. For instance, predictive analytics can forecast disease outbreaks, enabling healthcare providers to implement proactive measures to manage these health crises.
2. Finance
Financial institutions utilize machine learning to detect fraudulent activities, assess credit risks, and optimize trading strategies. These algorithms analyze historical transaction data to identify unusual patterns, which helps in flagging potentially fraudulent transactions in real-time. Moreover, predictive models guide investment decisions by analyzing market trends and consumer behaviors.
3. E-Commerce
E-commerce platforms leverage machine learning to enhance user experiences and boost sales. Recommendation systems powered by machine learning analyze consumer behavior to suggest products tailored to individual preferences, thereby increasing conversion rates. Additionally, chatbots utilizing natural language processing provide instant customer support, further enhancing customer satisfaction.
4. Manufacturing
Machine learning is revolutionizing manufacturing through predictive maintenance and quality assurance. By analyzing data from machinery, businesses can predict equipment failures before they occur, reducing downtime and maintenance costs. Moreover, machine learning aids in quality control by identifying defects in products using advanced imaging techniques and statistical analysis.
Challenges in Implementing Machine Learning
Despite the promising benefits, organizations face several challenges when integrating machine learning into their operations:
- Data Quality: The success of machine learning algorithms heavily relies on data quality. Organizations must ensure that their data is accurate, complete, and unbiased to avoid flawed predictions.
- Skilled Workforce: There is a significant demand for professionals skilled in machine learning and data analysis. Organizations often struggle to find qualified personnel to manage and implement machine learning initiatives.
- Integration with Existing Systems: Many businesses operate with legacy systems, making it challenging to integrate machine learning solutions seamlessly.
- Ethical Concerns: The use of machine learning raises ethical issues, particularly regarding privacy and data security. Organizations must navigate these concerns responsibly to maintain consumer trust.
Future Trends in Machine Learning for Businesses
As technology continues to evolve, several trends are likely to shape the future of machine learning in business:
- Automated Machine Learning (AutoML): AutoML tools will simplify the process of building and deploying machine learning models, making it more accessible to non-experts.
- Edge Computing: As more devices connect to the internet, machine learning will shift toward edge computing, enabling real-time data processing closer to the source, reducing latency and bandwidth usage.
- Explainable AI: There is a growing need for transparency in machine learning algorithms, where businesses will prioritize explainable AI to understand how decisions are made.
- Ethical AI Practices: Organizations will increasingly focus on implementing ethical AI practices, prioritizing fairness, accountability, and transparency in their machine learning models.
Conclusion
The integration of machine learning into business operations represents a paradigm shift that can unlock unprecedented potential for innovation and growth. This blog on machine learning has outlined its significance, diverse applications, and the challenges organizations might face. As businesses embrace this forward-thinking technology, they will not only stay competitive in their respective markets but also pave the way for a future driven by data and intelligent insights.
In order to thrive, organizations must continue investing in their machine learning capabilities, ensuring their teams are well-equipped to harness the power of machine learning and drive substantial business outcomes.